Sure, But What Does It Predict?
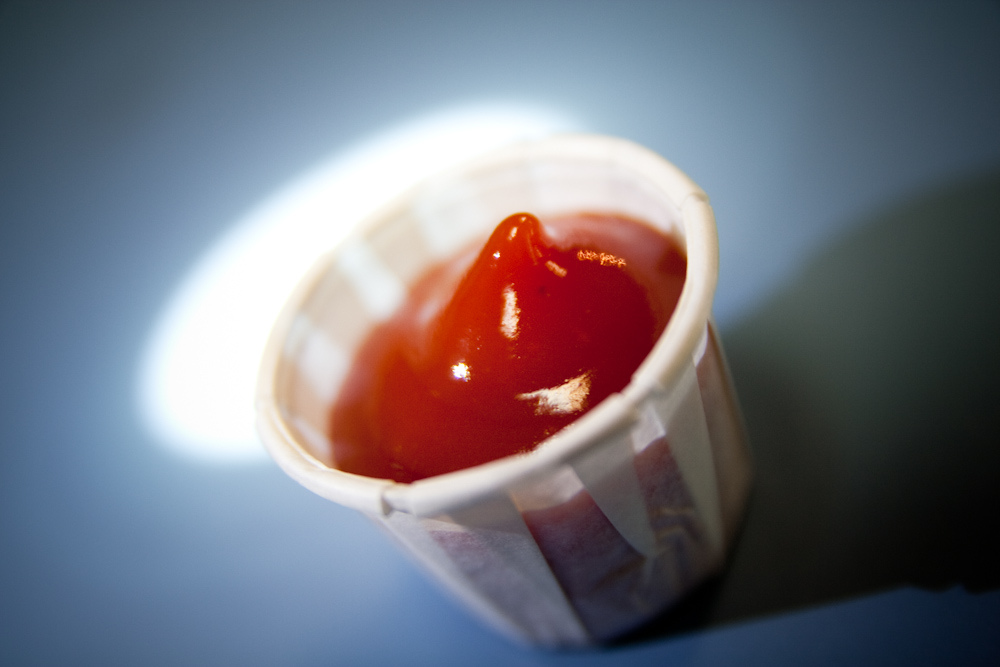
Ketchup is gross
He takes a bite of his bacon cheeseburger, “Medical providers don’t know how science works.”
“Tell me about it!” I’m having dinner in a noisy sports bar in the Midwest with an applied mathematician with ties to Fermilab. “Even the most basic concepts, like falsifiability, are lost on them.”
“I know!” He takes another bite.
I continue, “They don’t get that a hypothesis is not scientific unless it is falsifiable!”
His eyes dart my way and an eyebrow goes up. “No. You’re wrong. That’s not how science works.”
My eyes dart back at him and squint, brow furrowing. The sports bar goes quiet and the TVs all freeze. Everyone turns to look at our staredown, their mouths mid-chew. A tumbleweed rolls silently across the table between us.
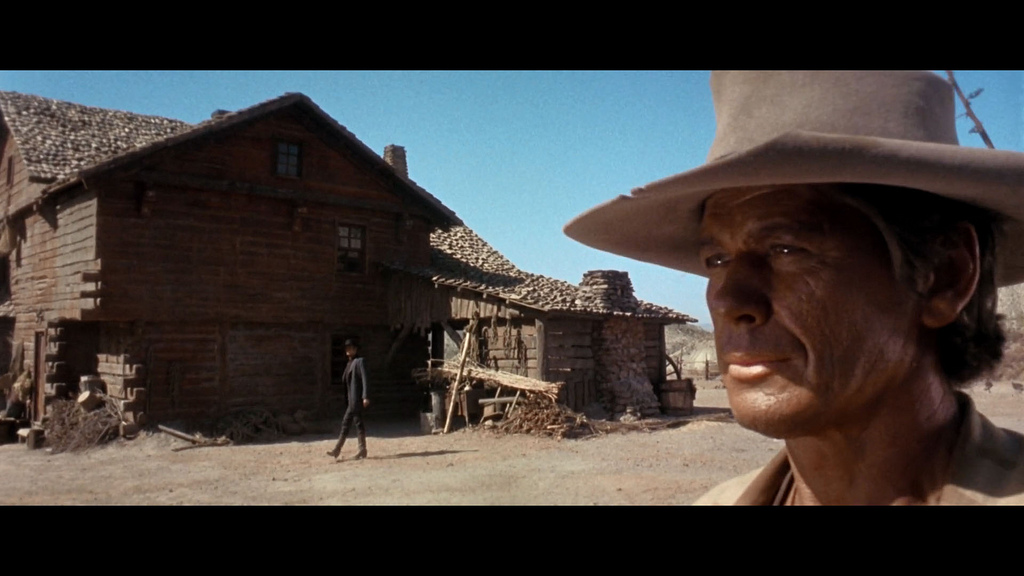
I’m…WRONG??? Let’s do this, punk!!!
Ok, maybe I’m overdramatizing this a little. But there was a moment of mental knuckle-cracking and I’m not entirely sure about the tumbleweed.
I state clearly, “Falsifiability is an established hallmark of science.”
“Yes, but your statement is inaccurate. You can’t falsify an idea directly.” He dips a French fry into his ketchup. I hate ketchup. Tomato, sugar, vinegar, gross. I’d rather put mayonnaise on my French fries, which is also gross.
“Sure you can! Through experimentation!”
“No. That’s not how it works.” He is starting to lose me. I’ve been here before. He’s going to drag out some tired, “How can we know anything? All we seek is plausibility,” metaphysical nonsense. Maybe as a mathematician he has gone all abstract on me…
He continues. “In order for a hypothesis to be considered scientific, it must be able to produce extremely precise PREDICTIONS that are themselves falsifiable.”
I start laughing. He is totally right. I concede immediately and we carry on with our original discussion. But what does this mean and why does it matter?
Hypotheses and theories are just prediction generating tools
First, a little defining of terms. A hypothesis is simply an idea that has not been explored experimentally. Through the process of experimentation, a hypothesis builds into a theory, which is the closest thing that we have to a “fact” in science. But keep in mind, a theory can never be proven “right”. As a matter of fact, theories inherently have some “wrongness”. Science accepts that it is absurd to think of any idea as being flawless or 100% “right”. Since this is counter-intuitive, let me make this crystal clear:
There is absolutely NO WAY that any idea is 100% “right”.
You have to remember that a theory is never complete. This is why they are constantly “under remodel”. The example I always give here is gravity. Newtonian physics predicts how gravity works. So does Einstein’s relatively. But neither are “right”. What can be said is that relativity is “less wrong” than Newtonian physics.
Relativity is damned good, really damned good, but gravity has been a little bit of a bugger when it comes to The Standard Model (particle physics). And that is good old gravity that we all know and love! At no point will we say, “All right then. That’s settled.”
Experimentation modifies and progresses a theory
So this is where prediction comes in. We can use theoretical logic all day long but that can lead in so many different directions. That is not to say it is wrong to exercise logic. It is actually imperative to sit and ponder ideas. But remember, the idea is just a tool. Once you develop a tool, you have to test it. If you generate a theoretical hammer, at some point you need to swing it, HARD, and see if it breaks in your hand.
As I mentioned before, hypotheses and theories are just prediction generating tools. So in order to use these tools, you must generate a prediction that can be tested. It will look something like, “If this idea was WRONG, then we should NOT see this result when we run the experiment.” Then you go look. Notice that I didn’t say, “If this idea was RIGHT…” You try to falsify; not confirm.
The more counter-intuitive and absurd the prediction the better
Now, I could hypothesize that the sky is generated by a Rainbow Farting Unicorn (Figure 1). My Rainbow Farting Unicorn hypothesis predicts that, due to atmospheric conditions filtering the farted rainbow, the sky should be colored blue. If the sky were anything other than blue, my hypothesis is wrong. Let’s go outside and look. Success!
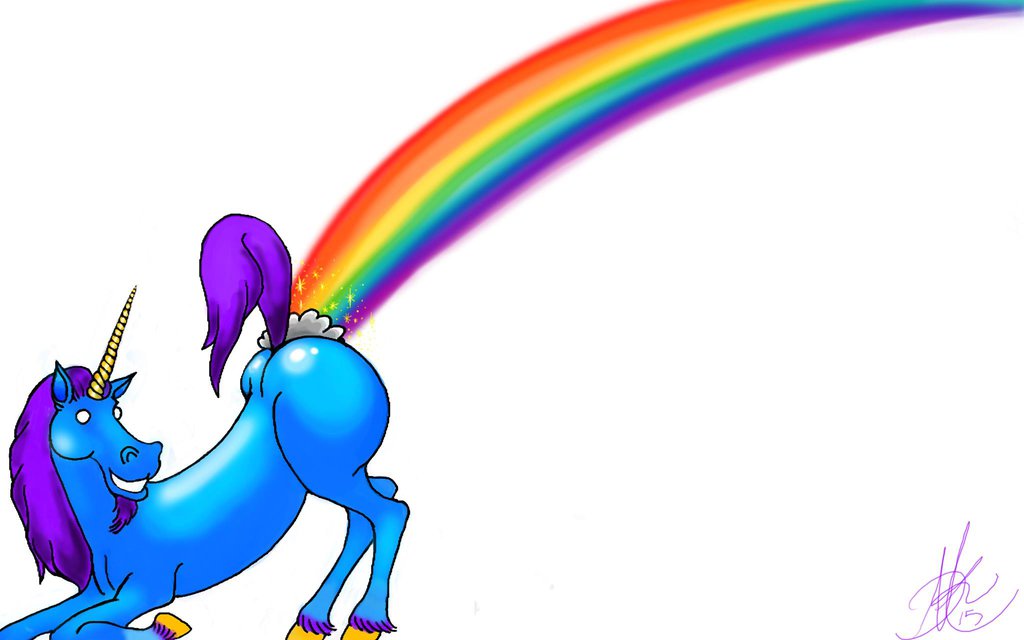
Figure 1
We know this is wrong, but why? This is where absurdity comes in. Now, while my hypothesis was absurd – “Rainbow Farting Unicorn” – the generated prediction was not – “Sky will be blue.” If the prediction is too generic, vague, or universal then it could be explained by almost anything. While a hypothesis will of course need to explain the obvious, in order for it to be tested it needs to predict something that nothing else does. Something that “shouldn’t be”. In other words, something that goes AGAINST what you would expect. Something that makes you say, “That’s funny…”
The most exciting phrase to hear in science, the one that heralds new discoveries
, is not “Eureka” but “That’s funny…”
– Isaac Asimov
Typically, that finding has not been seen in the past, but only because no one has looked for it. The fact that it has been weirdly predicted by a hypothesis is what makes you go looking for such oddly specific findings. Confused? Let’s look at an example…
Light is a particle AND a wave
In 1799, there was a problem. Light, shown by Newton in the 17th century to be a particle (the photon), acted weirdly in an experiment where it was passed through a double slit. Instead of creating two distinct light patterns as expected, it created interference patterns consistent with the action of waves. This created the hypothesis that light was not only a particle, but also a wave. So, as is the way in science, scientists went off to create testable predictions from that hypothesis to see how useful the idea might be.
In 1818, Siméon Poisson came up with a prediction. If you shot a tightly focused beam of light at a spherical object, it would cast a shadow of the object on the wall behind it (duh). The double slit experiment predicted that there would be some evidence of interference around the edges of the shadow, but Poisson’s calculations based off the wave hypothesis predicted that there should also be a focal point of light in the dead center of the shadow which is completely counter-intuitive and absurd. It is so counter-intuitive that Poisson famously used the resulting logical absurdity to outright reject the hypothesis. He refused to even run the experiment because it was so ludicrous. Of course, when someone by the name of François Arago did…
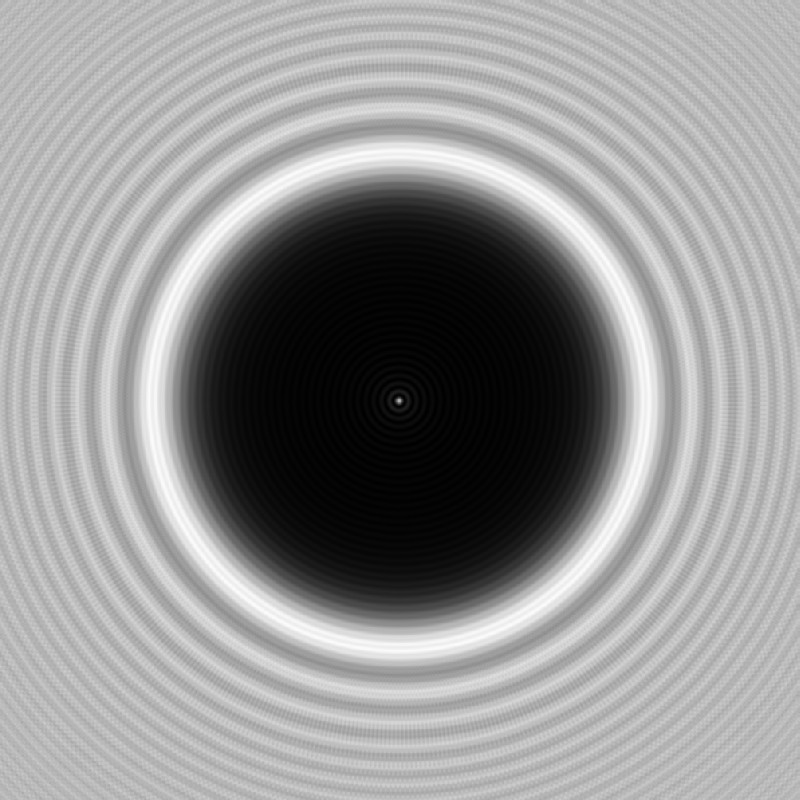
Arago Spot – look in the very center of the shadow.
…the spot presented itself. The absurd prediction fails falsification after all.
[For more info on this, see this very informative post by Ethan Siegel on his blog, Starts With a Bang.]
So why does this matter?
It matters because too many medical providers get into this mindset of, “Well, it hasn’t been proven wrong.” And then they hold it up to a vague prediction: “The patient will feel better.” Dammit people! That is just like my Rainbow Farting Unicorn – which has not been proven wrong and explains why the sky is blue. That doesn’t make it “right”.
Some hypotheses (like my Rainbow Farting Unicorn) don’t produce ANY useful predictions. That simply makes such ideas NOT scientific (which makes no comment as to whether an idea is true or false).
Your hypothesis should produce a unique, specific, and precise prediction that works wherever you apply it. Again, “If this had some truth to it, then when we look over here we should see this very specific result. And when we look in this completely other place the hypothesis predicts this other very specific result, and if we were to look over there…” and so on.
If you find ANYTHING other than the predicted results, you have falsification – an error to the experiment. Either the experiment was flawed, or the hypothesis was. Period. The less that it predicts experimental findings, the more wrong or incomplete the hypothesis and the greater the need to reexamine. This is not a bad thing, that is how ideas evolve.
The best we can ever say is, “This theory has produced some very specific, precise, and useful predictions that were not wrong. We should continue using it to generate more.”
In summary…
- Ketchup is gross
- Hypotheses and theories are tools to generate predictions
- Testing the accuracy of precise predictions is how ideas are refined
- Rainbow Farting Unicorns are cool
The featured image on this post is “Ketchup Cup Macro” by Steven Depolo via Flickr.
The “I’m…WRONG???” image on this post is “showdown in cinecitta technicolor” by Karl-Ludwig Poggemann via Flickr.
“Figure 1” image on this post is “Rainbow Manufacturing” by Ooh-A-piece-of-Candy via Deviant Art.
The “Arago Spot” image on this post is “Poissonspot simulation d4mm” by Treisinger via Wikicommons.
Looking for the comments section? Learn why you can’t find them.